The applications of machine learning, as an important part of technology, are evolving rapidly. We use machine learning in our daily lives unconsciously, such as Google Maps, Google Assistant, Alexa, and so on. The need for machine learning engineers is in high demand due to evolving technology and the production of huge volumes of data called Big Data. On average, an ML engineer can earn 111,490 USD. Therefore, in this article, we want to introduce you to a number of applications of machine learning. You can also access the machine learning article.
1- Virtual personal assistant:
There are various virtual personal assistants such as Google Assistant, Alexa, Cortana, Siri. As the name implies, they help us find information using voice training. These assistants can only help us in various ways with voice instructions such as playing music, calling someone, opening emails, scheduling appointments, and so on. Recently, personal assistants have been used in chat bots, which are implemented in various food ordering programs, online educational websites, as well as in travel programs. Machine learning is an important part of these personal assistants because they collect and modify information based on their previous involvement with users. Later, this data set is used to provide results that are in line with users’ preferences.
Virtual assistants are integrated with a variety of platforms. For example:
- Smart speakers: Amazon Echo and Google Home
- Smartphones: Samsung Bixby on Samsung S8
- Mobile applications: Google Allo
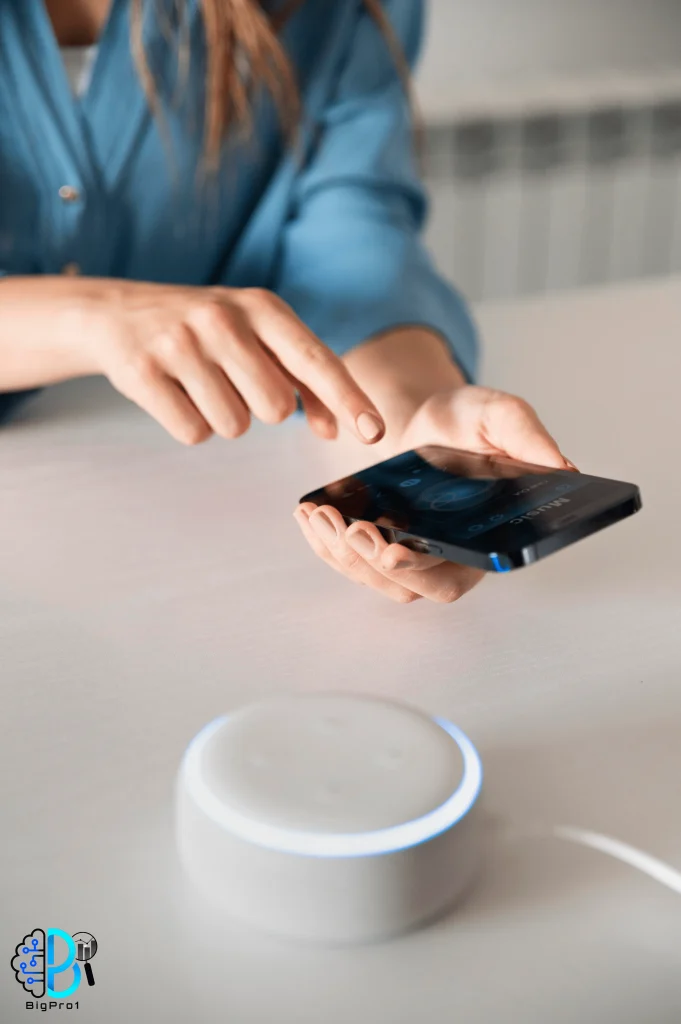
2- Transportation:
Traffic forecasting:
We have all used GPS navigation services. As we do this, our current locations and speeds are stored on a central server for traffic management. This data is then used to map current traffic. Machine learning in such scenarios helps to estimate areas where congestion can be found based on daily experiences.
Online Transport Networks:
Online Transport Networks: When booking a taxi, the program estimates the price of the trip. How to minimize deviations when sharing these services? The answer is machine learning. Jeff Schneider, Uber ATC’s chief engineering officer, reveals in an interview that they use ML to define hours of price increases in anticipation of rider demand. Throughout the service cycle, ML plays a key role.
3- Image classification and image recognition:
Businesses also turn to machine learning, deep learning, and neural networks (a set of algorithms designed to detect patterns) to help them understand images. Image recognition is one of the most common applications of machine learning that is used to identify objects, people, places, digital images and so on. A common use of image recognition is to suggest automatic tagging of friends. Facebook uses face recognition to automatically find the face of a person who matches its database, and therefore suggests that we tag that person based on DeepFace. The Facebook DeepFace project is responsible for recognizing faces and identifying people in the image. It also provides Alt tags (alternative tags) for images that have already been uploaded to Facebook.
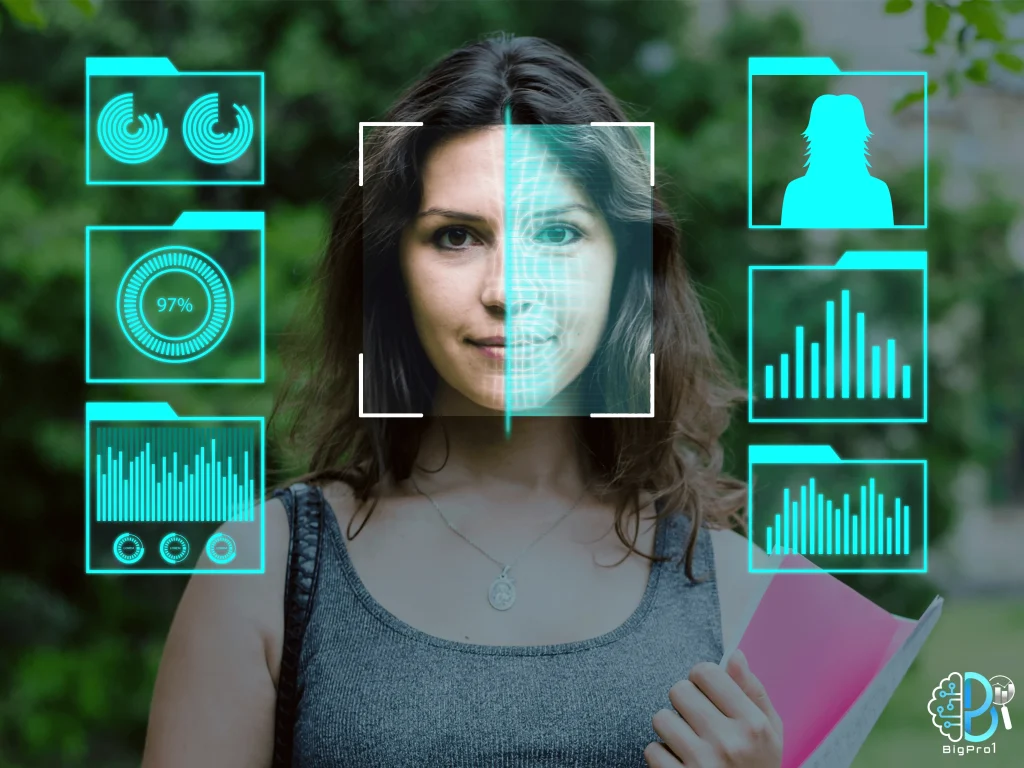
4- Spam filter and email malware:
Every time we receive a new email, it is automatically filtered as important, normal and spam. We always receive an important letter in our inbox with the important symbol and spam emails in our spam box, and the technology behind it is machine learning. Here are some spam filters used by Gmail:
Content filter, header filter, public blacklist filter, rule-based filters, license filters
Some machine learning algorithms such as multilayer perceptron, decision tree and Naïve Bayes classifier are used to filter email spam and detect malware.
More than 325,000 malwares are detected daily, and each code is 90 to 98 percent similar to its predecessors. System security programs designed by machine learning understand the coding pattern. Therefore, new malware can be easily detected and protected against changes of 2 to 10%.
5- Online fraud detection:
Machine learning is proving its potential to make cyberspace a safe place, and tracking online money scams is one example. Fraud detection is one of the most essential applications of machine learning. Whenever a customer makes a transaction – the machine learning model completely photographs his profile to look for suspicious patterns.
For example: Paypal uses ML to protect against money laundering. The company uses a set of tools to help them compare millions of ongoing transactions and differentiate between buyer and seller. In machine learning, problems such as fraud detection are often considered classification problems.
6- Product recommendation:
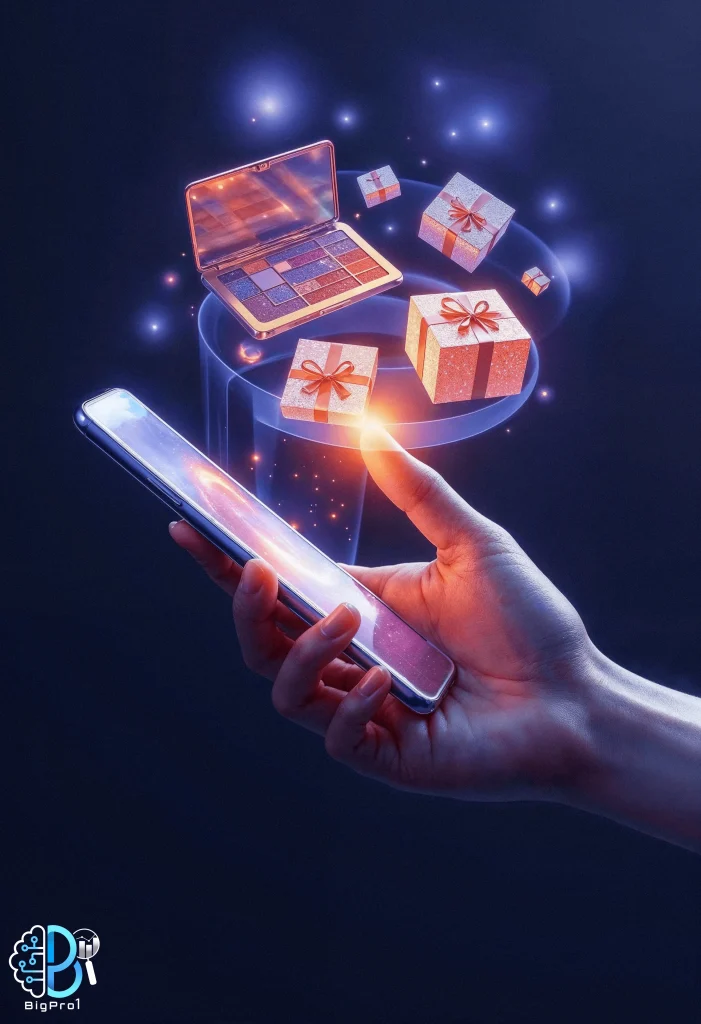
Machine learning is widely used by various e-commerce and entertainment companies such as Amazon, Netflix, etc. to recommend a product to the user. Suppose you review a product on Amazon, but then you will not buy it. But the next day, you’re watching videos on YouTube and suddenly you see an ad for the same thing. You switch to Facebook, you see the same ad there. So how does this happen?
Well, this is because Google tracks your search history and recommends ads based on your search history. This is one of the most interesting machine learning programs. In fact, 35% of Amazon’s revenue comes from product recommendations.
Likewise, when we use Netflix, we find recommendations for entertaining series, movies, etc., and this is done with the help of machine learning.
7- Self-driving automobile:
One of the most interesting applications of machine learning is self-driving automobile. Machine learning plays an important role in self-driving cars. Tesla, the most popular car company, is working on self-driving cars. It uses unsupervised learning to teach car models to recognize people and objects while driving.
Last word:
Machine learning is the combination of code development with data, which is defined as a predictive model for obtaining output or discovering trends. When ML is combined with deep learning, neural networks, computer vision, and big data, it has tremendous potential to change any segment and enhance the customer experience, so in this article we found that mentioned applications and much more, make machine learning enable to play a very important role in human daily life; In such a way that most of the time we use it unconsciously.